AI is no longer a futuristic concept—it’s now a key driver for businesses across the globe. In 2024, the AI market grew beyond $184 billion, with countless companies relying on it to streamline processes and drive innovation. However, as AI becomes more embedded in business strategies, it’s essential to consider the full cost.
AI costs include the technology and infrastructure, the skilled professionals in charge of running it, and the ongoing management to keep everything on track. AI solutions come in all shapes and sizes, from custom-built platforms designed specifically for your business to ready-made tools like chatbots from third-party providers.
Custom solutions can cost hundreds to thousands of dollars, while pre-built options, depending on their complexity, charge a yearly fee.
So, let's break down the AI development costs your organization should be aware of.
Factors Influencing AI Costs
Implementing AI in an organization involves various cost factors that vary significantly based on the project's specific needs.
Below are some key elements that can influence the overall cost of AI solutions.
Project Scope and Complexity
The scope and complexity of your AI project are major cost drivers. A simple data science project can be developed without specialized hardware, but complex practical applications, such as a real-time object detector, will require expensive GPUs for training. Larger projects often demand more time, specialized expertise, and higher levels of infrastructure, all of which contribute to increased costs.
For example, a sales forecasting model can often be trained on a regular CPU. Still, modern Generative AI projects require tens of high-end GPUs and dedicated teams for data cleaning and labeling.
Data Quality and Availability
AI systems rely heavily on data, and the availability and quality of that data significantly impact the overall cost. Gartner reports that every year, organizations lose $12.9 million due to poor data quality. Clean, well-organized data minimizes preprocessing costs. However, incomplete or messy data can increase expenses due to the additional time and resources needed for data collection, cleaning, and labeling.
Poor data quality can lead to longer development cycles as the AI system struggles to achieve accuracy. This can further inflate costs.
Customization Needs
The level of customization required also plays a key role in determining the cost of an AI solution. Off-the-shelf AI tools such as Hugging Face Transformers can be more affordable, as they are designed for broader use cases and require minimal adjustments.
On the other hand, custom-built AI systems tailored to meet your organization's specific needs often come with higher development and integration costs. This is because they require specialized development, custom algorithms, and ongoing maintenance.
AI Features
The features you choose for your AI solution will directly affect its cost. Advanced functionalities such as natural language processing (NLP), real-time decision-making, or predictive analytics add to the overall expense.
For example, building real-time dashboards and data streaming pipelines for business decision-making requires additional investment in data engineers, analysts, and hosting platforms. Similarly, creating and deploying MLOps pipelines for managing machine learning models adds to the overall cost.
Scalability Requirements
Your organization's scalability needs can greatly influence ongoing AI costs. Small-scale implementations may have lower upfront and operational costs. However, suppose you need your AI solution to handle large-scale data processing or enterprise-level deployments. In that case, the costs will increase due to the need for more robust infrastructure, storage, and computing power.
Investing in scalable infrastructure ensures your AI solution can grow with your business but requires more significant investment initially.
Estimating AI Development Costs
When it comes to AI, the total cost of development can vary widely depending on the scale, complexity, and specific needs of your project. From infrastructure and labor to software, training, and operational costs, each element adds up.
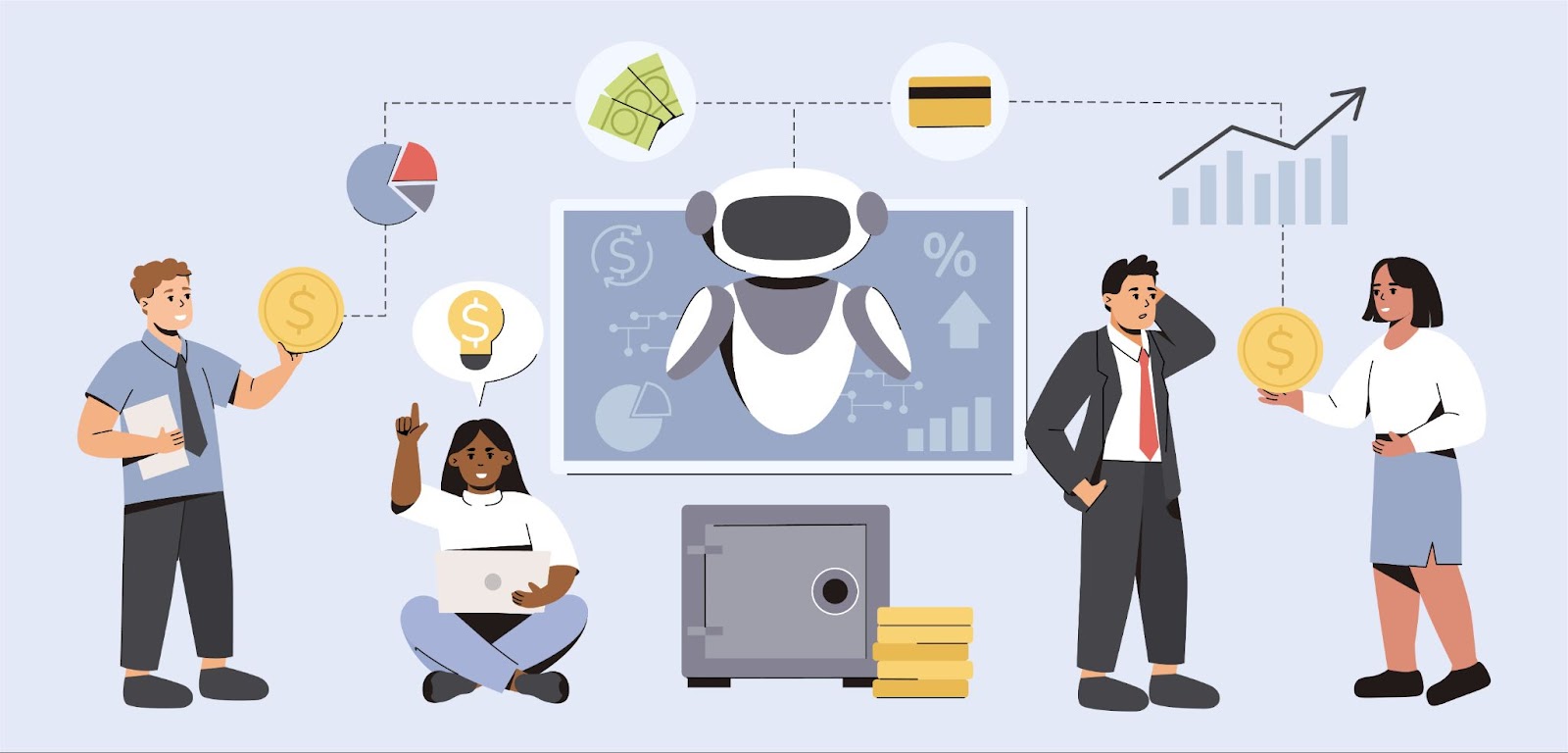
On average, businesses can expect to invest between $10,000 to $49,999 or more in AI development.
Below is a breakdown of the key components and their estimated costs.
Infrastructure Costs
AI projects require specialized hardware like graphics processing units (GPUs) and tensor processing units (TPUs) for handling data and complex computations. High-end GPUs can cost more than $10,000 each, with larger projects needing thousands for training. Renting is often more cost-effective, with the price for NVIDIA T4 GPU starting from $0.35/GPU in the Google Cloud Platform.
Many businesses opt for cloud platforms like AWS, Google Cloud, or Azure to avoid high upfront hardware costs. Cloud solutions offer scalability but come at a price. Depending on the number of users and the frequency of experimentation and training, the monthly costs can be between a few hundred to several thousand dollars.
On-premise solutions require significant investment but may be more cost-effective for long-term, large-scale operations.
Labor Costs
The salaries for AI professionals are a significant part of the total cost. For key roles:
- Data Scientist: Average base salary of $122,635/year.
- Machine Learning Engineer: Average base salary of $162,082/year.
- AI Developer: Average base salary of $129,348/year.
Depending on the team's size and the project's complexity, businesses can expect to invest heavily in securing skilled talent. For a team of just three experts (one from each role), the combined annual cost would exceed $400,000.
Additionally, end-to-end AI projects often require data engineers to create and maintain robust data pipelines, which adds to the overall costs. This ensures that machine learning engineers have reliable and scalable access to data.
Moreover, upskilling current staff to work with AI tools and technologies is often necessary. The cost of professional certifications can be a few hundred dollars per employee, depending on the complexity and duration of the training. For example, the Azure AI fundamentals certification costs $99, and the cost increases for more advanced courses.
Conversely, many companies hire AI consultants for specialized tasks or temporary expertise. Consulting fees typically range from $200 to $400 per hour. Depending on the scope and duration, a project's total cost can range up to tens of thousands of dollars.
Software and Tooling Costs
Acquiring specialized AI platforms, tools, and software licenses can vary greatly depending on the business's needs. An AI project will require access to various platforms and tools for data storage, dataset annotation and curation, model training, and deployment. Some providers like Picsellia offer all such features in a single package. The costs can start from a few hundred dollars to a few thousand, depending on the number of users and the pricing tier selected.
Moreover, organizations may opt for selective services such as just an annotation tool to create a dataset. In this case, the costs will be considerably lower but with an inconvenient development experience and possibly increased development time.
With such a wide range of options available, businesses can expect software and tooling costs to range from a few hundred dollars per month for smaller applications to thousands of dollars for high-volume usage.
Deployment and MLOps Cost
Once the model is trained, it is often served on cloud platforms as an API. This incurs ongoing costs based on API call volume, which can add up quickly. Serving a basic machine learning model on AWS typically involves variable costs depending on usage and the specific services utilized.
However, Amazon SageMaker offers a free tier for new users, allowing you to get started at no cost. For on-demand pricing, you can use the AWS Pricing Calculator.
Moreover, maintaining an MLOps pipeline adds to these costs, as it involves constant monitoring and retraining of models, requiring data engineers and scientists, which increases labor costs and uses cloud resources.
While MLOps pipelines are essential for model performance, they come with recurring personnel expenses, cloud usage, and data acquisition expenses.
Security Costs
Ensuring that AI systems comply with data privacy regulations (e.g., GDPR, HIPAA) and are secured against potential cyber threats adds to the overall expenses. Depending on the level of compliance required, businesses may need to invest in security audits, certifications, and legal consultations. These expenses vary widely based on the project's scope and the specific regulatory requirements, ranging from a few thousand dollars to significant amounts for complex implementations.
Other Costs
Managing an AI project includes coordination between teams and leadership. The costs will vary depending on the team's size, project complexity, and the level of management required.
Furthermore, running AI models, particularly large-scale ones, can significantly increase energy bills. These operational costs fluctuate based on the scale of the models and the resources required to run them.
Tips to Optimize AI Costs/ Cost-Effective Strategies for AI Implementation
Managing AI costs is necessary to ensure a successful implementation without overspending.
Here are some practical strategies to help keep expenses under control:
- Outsource non-core AI development: Consider outsourcing non-core AI development tasks rather than building everything in-house. This allows your team to focus on critical areas while reducing costs by using external expertise for less essential components.
- Build in-house models where possible: Low-code/no-code tools and platforms can be expensive, especially during long development and training cycles. Building in-house models using open-source frameworks like Tensorflow and Pytorch can significantly cut down costs.
- Use pre-trained models: Instead of developing models from scratch, use pre-trained models for common tasks like image recognition or natural language processing. Platforms like Hugging Face or Google Cloud AI offer a wide variety of pre-built models, which can save time and reduce development costs.
- Adopt cloud-based AI solutions: Cloud-based AI platforms like AWS AI or Microsoft Azure AI let you scale as needed without having to invest in costly infrastructure. You only pay for what you use, making it a flexible, cost-efficient option.
- Monitor and optimize AI workload: Regularly monitor and optimize your AI workloads to avoid unnecessary expenses. For instance, If your models don’t need as much processing power or storage, you can scale back and save money in the long run. You can also apply monitors and alerts on cloud platforms to avoid unnecessary cost leakage.
Optimize AI Costs with Picsellia's Complete MLOps Platform
AI projects can be expensive, especially when it comes to hardware, data management, and skilled talent. These costs can quickly add up for companies working on computer vision projects. However, with the right tools and strategies, these expenses can be optimized without sacrificing quality or performance.
Picsellia is a complete MLOps stack for computer vision which provides you with a cost effective all-rounder tool for your vision AI project.
Keep your vision AI projects on budget while still achieving great results with Picsellia.
Get a free demo today to learn how Picsellia can help cut AI costs and enhance your computer vision projects.