IBM defines computer vision as “a field of artificial intelligence (AI) that uses machine learning and neural networks to teach computers and systems to derive meaningful information from digital images, videos, and other visual inputs—and to make recommendations or take actions when they see defects or issues.”
It falls under the umbrella of AI which extracts information from images. As AI gains momentum, so does computer vision in several industries. Today, computer vision is being used in everything – from drone technology to inspecting production lines in manufacturing.
But who are the ‘Early Adopter Companies’ of Computer Vision?
(NOTE: An ‘early adopter’ is any person/organization who starts using a product or service as soon as it becomes available. They are often seen as risk bearers of innovations. They are more popular in the tech industry due to their willingness to adopt and try new technologies, which helps further advance software.)
Information Technology Companies
Google:
Google is often seen as a ‘pioneer’ in the field due to its massive contribution to the development of this software. A brief look at Google’s computer vision journey:
- 2009 - ‘Google Goggles’, a one-of-its-kind computer vision project launched as an image recognition application by Google. Consumers could now search the world wide web using the pictures they captured via their mobile cameras. It could recognize landmarks, and logos and solve sudoku puzzles.
- 2014 - The acquisition of DeepMind, a leadering AI research company which significantly impacts Google’s capabilities in Machine Learning and Computer Vision. This move is strategic for Google to develop its own advanced vision algorithms.
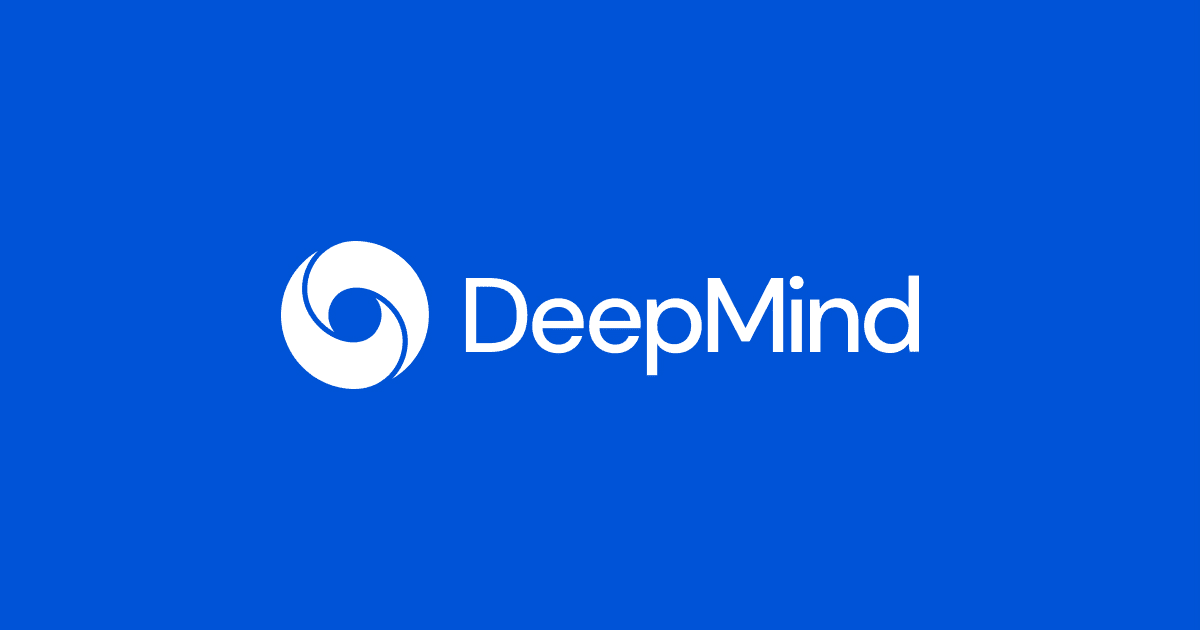
- 2015 - Google Photos is launched which marks a milestone in Google’s computer vision journey. This application uses advanced image recognition algorithms to automatically organise and tag photos, making them searchable.
- The same year, Google open-sourced its machine learning library ‘TensorFlow’, which has now become a common tool for developing computer vision applications. This move has helped normalize access to advanced AI technologies.
- 2016 - Google introduces Cloud Vision API which makes its computer vision capabilities available to developers worldwide. This API enables features like labeling, face detection, and optical character recognition (OCR).
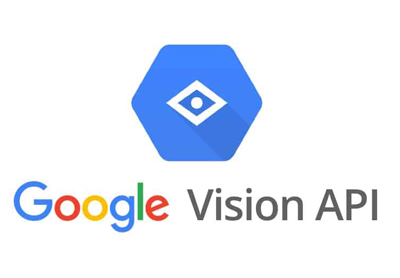
- 2017 - Google Lens is created which represents a milestone in mobile computer vision. This allows users to search and interact through their smartphone cameras by identifying objects, translating texts, and providing information about landmarks.
- Google also integrates computer vision into applications such as Google Maps (Live View navigation) allowing users to navigate streets in real time.

Meta (Facebook)
Meta (formerly Facebook) has been a significant player in computer vision since the early 2010s, starting with basic facial recognition for photo tagging. One of its earlier milestones includes the introduction of DeepFace in 2014, achieving near-human accuracy in face verification. The acquisition of Oculus VR that same year showed Meta's commitment to virtual and augmented reality, areas heavily dependent on computer vision. The establishment of Facebook AI Research (FAIR) in 2015 led to innovations like the Lumos image understanding platform and the open-source PyTorch library. Recent years have seen Meta focusing on enhancing AR capabilities in its apps, developing technologies for the metaverse, and improving content moderation through advanced image and video analysis. Throughout its history, Meta has consistently pushed the boundaries of computer vision, viewing it as crucial for the future of social interaction and digital experiences.
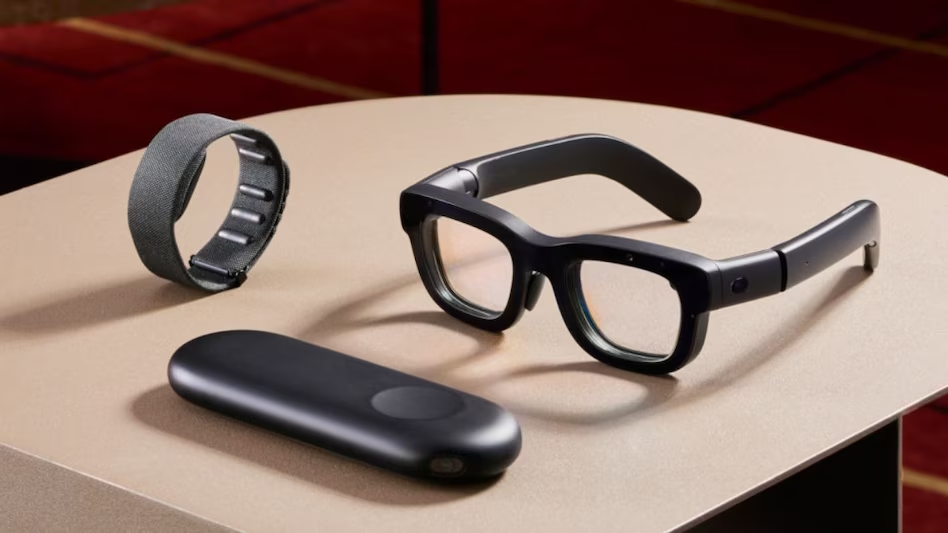
Amazon
Amazon's journey in computer vision began in the early 2010s with efforts to improve product image recognition for its e-commerce platform. In 2015, Amazon introduced Amazon Rekognition, a powerful computer vision service that can identify objects, people, text, scenes, and activities in images and videos. This marked a major step in making advanced computer vision capabilities accessible to developers and businesses. Amazon's commitment to computer vision expanded with the launch of Amazon Go stores in 2018, which use computer vision, sensor fusion, and deep learning to enable a cashierless shopping experience. The company has also integrated computer vision into its warehouse operations for inventory management and quality control. In recent years, Amazon has continued to innovate in the field by developing Amazon SageMaker, their fully-managed machine learning platform, to support the development of custom computer vision models. The company has also introduced AWS DeepLens, a deep learning-enabled video camera for developers to build and deploy computer vision applications. Amazon's computer vision technologies are used for enabling intelligent search, content management and analysis, personalized services, and gaining customer insights. The company continues to invest heavily in this technology, recognizing its potential to transform various aspects of business and daily life.
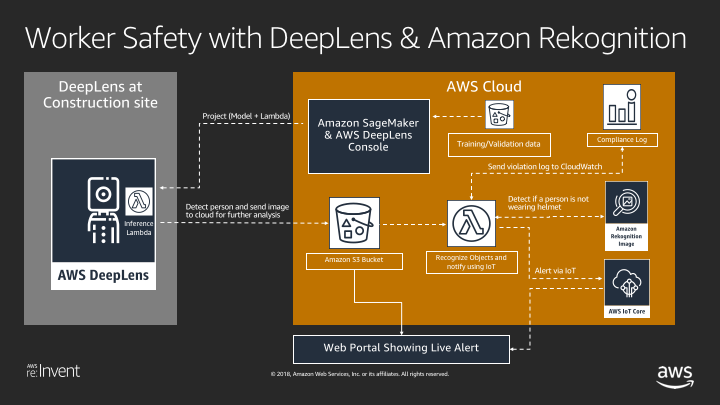
Manufacturing & Industrial Companies
General Electric (GE)
General Electric (GE) has integrated computer vision technologies into various aspects of its operations, focusing on improving efficiency, quality control, and safety in industrial settings. In manufacturing, GE has implemented computer vision systems for:
- Quality control and inspection of products on assembly lines
- Monitoring and analyzing equipment performance in real-time
- Enhancing worker safety by detecting potential hazards or unsafe conditions
GE has also applied computer vision in its power generation and aviation divisions, using the technology for tasks such as:
- Inspecting power plant equipment and infrastructure
- Analyzing aircraft engine components for wear and potential failures
Throughout its history, GE has consistently invested in research and development of computer vision technologies, recognizing its potential to transform various industries. The company continues to explore new applications of computer vision across its diverse portfolio of businesses, aiming to improve operational efficiency, product quality, and customer experiences.
Orbital Insight
Orbital Insight, founded in 2013 by James Crawford, is a geospatial analytics company that has been at the forefront of applying computer vision and machine learning techniques to satellite imagery. Orbital Insight was established with the goal of combining commercial and government satellite images with advanced artificial intelligence systems to extract valuable insights. The company's first project involved analyzing the health of corn crops using computer vision techniques.By 2017, Orbital Insight had developed sophisticated algorithms to extract insights from satellite imagery, including analyzing car counts in parking lots to predict retail trends and measuring oil storage levels by examining shadows in oil tanks. The company also began working with the US Department of Defense to improve imagery applications in poor weather conditions. In 2019, Orbital Insight released its GO platform (later renamed TerraScope), which allowed customers to directly search and analyze satellite imagery and geolocation data. This marked a significant step in making computer vision technology more accessible to a broader range of users. The company has developed capabilities to analyze various types of data, including satellite imagery, drone footage, and cell phone geolocation data, to provide insights across multiple industries such as retail, energy, agriculture, and defense.
Automotive
Tesla
In the early 2010s, Tesla began integrating computer vision into its vehicles, initially focusing on basic driver assistance features. The company's Autopilot system, introduced in 2014, relied heavily on computer vision for tasks like lane keeping and adaptive cruise control. In 2016, Tesla announced that all new vehicles would be equipped with hardware capable of full self-driving, including multiple cameras for 360-degree vision. This marked Tesla's commitment to a vision-based approach to autonomous driving. In 2017, Tesla introduced its Neural Net for Autopilot, an AI system that processes visual data from the car's cameras. This system, which Tesla calls "Tesla Vision," forms the core of their autonomous driving capabilities.
By 2021, Tesla began transitioning away from radar sensors, relying solely on cameras and neural networks for Autopilot and Full Self-Driving (FSD) features. Tesla has continued to refine its computer vision capabilities, introducing features like traffic light and stop sign recognition in 2020. The company's AI team, led by Andrej Karpathy until 2022, has been instrumental in developing advanced neural networks for tasks such as depth estimation, object detection, and road layout prediction. Throughout its history, Tesla has leveraged its large fleet of vehicles to collect vast amounts of real-world driving data, which is used to train and improve its computer vision algorithms. The company has also developed custom AI hardware, including the Full Self-Driving (FSD) computer, to process the complex visual data required for autonomous driving. Tesla's approach to computer vision continues to evolve, improving the capabilities of its vehicles and pushing the boundaries of vision-based autonomous driving systems.
General Motors (GM)
General Motors (GM) has been integrating computer vision technology into its vehicles and manufacturing processes for over a decade. In the early 2010s, GM began incorporating driver assistance features that relied on computer vision, such as lane departure warnings and forward collision alerts. The company advanced its computer vision capabilities with the introduction of Super Cruise in 2017, a hands-free driving assistance system that uses high-definition maps, high-precision GPS, and a driver attention system with eye-tracking technology. In recent years, GM has expanded its use of computer vision in manufacturing, implementing AI-powered quality control systems to detect defects on assembly lines. The company has also been developing more advanced autonomous driving technologies through its Cruise subsidiary, which heavily relies on computer vision for object detection and navigation. GM continues to invest in computer vision research and development, viewing it as a crucial technology for the future of automotive safety, autonomous driving, and smart manufacturing.

Retail
Walmart
Walmart has been an early adopter of computer vision technology in retail. In the mid-2010s, Walmart began experimenting with computer vision for inventory management and customer experience improvements. In 2017, the company started testing computer vision-powered robots to scan shelves for out-of-stock items, incorrect prices, and misplaced products. By 2019, Walmart had deployed these robots in over 1,000 stores. In 2022, Walmart Canada announced a major rollout of an automated vision system to keep store shelves stocked, using AI-based software from Focal Systems to automate out-of-stock detection. The system uses cameras mounted on shelves to scan barcodes and QR codes every hour, triggering replenishment orders when necessary. Walmart has also implemented computer vision in its Sam's Club stores, introducing a "Scan & Go" feature that allows customers to skip checkout lines. In 2024, the company revealed plans to use AI and computer vision to eliminate queuing at Sam's Club exit areas. Throughout its history with computer vision, Walmart has focused on improving operational efficiency, improving their customer experience, and gathering valuable data on shopping behaviours.

Home Depot
Home Depot's journey with computer vision began in the late 2010s as part of its digital transformation efforts. In 2017, the company partnered with Hover, a startup that uses computer vision technology to create 3D models of homes from smartphone photos. This partnership allowed Home Depot customers to visualize home improvement projects more easily. In recent years, Home Depot has expanded its use of computer vision in various areas of its business. The company has implemented computer vision-based systems for inventory management, using cameras and AI to track stock levels and identify misplaced items. Home Depot has also explored using computer vision for enhancing the in-store customer experience, such as developing mobile apps that use image recognition to help customers find products or get information about items they see in the store. Additionally, the company has invested in computer vision technology for loss prevention, using AI-powered cameras to detect potential theft and improve store security. As Home Depot continues to innovate, computer vision remains a key technology in its efforts to improve operational efficiency and provide better services to its customers.
These early adopters have paved the way for wider implementation of computer vision across various sectors, proving its potential for innovation. As the computer vision continues to advance, we can expect to see even more companies integrating computer vision into their products and services.
To learn more about how computer vision can benefit your company and help you make more efficient business choices, book your personalised demo with Picsellia.