High turnover rates are real. There are many reasons behind people’s growing tendency to change teams, such as career changes, internal migrations, gap years, etc. The days when people used to remain in the same company forever are gone…
Nowadays, companies wanting to stay ahead of the competition must be able to accept this phenomenon and be prepared for it. A wide part of such preparation involves having a reliable onboarding plan.

Today we are going to focus on the onboarding process of machine learning and data science teams. We believe it is even harder to ensure a consistent workflow for these kinds of jobs. Well, it’s also the field we know the best!
Why is the on-boarding of ML collaborators harder than others?
The uniqueness of the ML team is that they usually deal with various dimensions:
- Data-driven business processes
- Explorations and experiments
- Data and data platforms
- Public/private clouds
- ML technology and models
Supporting these dimensions requires various dedicated teams such as product owners, business analysts, data analysts, data scientists, ML engineers, data engineers, software engineers, and DevOps engineers.
Walking a new employee through all these areas can be a real pain, giving them access to all the tools, data storages and ML models.
The worst part of all this is that you will need to do it quite often...
Due to the fast-changing technology landscape, severe market shortages, opportunities to combine multiple roles into one, etc., teams are choosing role/team transitions at a faster rate than other roles in other types of team software products.
In our personal observations, we see that this happens at least once every three to six months. Anticipating similar ratios, we have seen that the best strategy is to focus on sharing knowledge and ownership, combined with a strong onboarding/exiting process.
"In our organization, there is a lot of changes in our Data science research teams, As a team leader I usually need to dedicate at least one day a week to the onboarding and formation of the new collaborators, that's a huge part of my work" Sophie, AI Team Lead
How can 🥑 Picsellia 🥑 facilitate your onboardings ?
One of the biggest pain points of onboarding talents in your ML team is the heterogeneity of tools and processes. At Picsellia, we believe that you shouldn't build a toolchain of 6 tools… that’s why we built 6 tools in 1 platform to help you make your processes as smooth as possible.
Our philosophy is based on 3 pillars:
- Traceability
- Reproducibility
- Observability
These pillars can sound a bit abstract so let’s translate them into questions…
- Where are all our experiments?
- How can I ensure that all my data is not outdated?
- How to give access to the company's previous work to my new employee?
Tracking your experiments is key for new employees

As you know, deep learning is mainly a try and error process, involving a lot of experimentation. When starting a project, it’s quite impossible to avoid this step, BUT once your team has done the first trials, you MUST be able to access them in order for your new employees to know what NOT to try! This will save your team a lot of time and your company a lot of money on cloud GPUs costs.
Always link your Datasets to your training
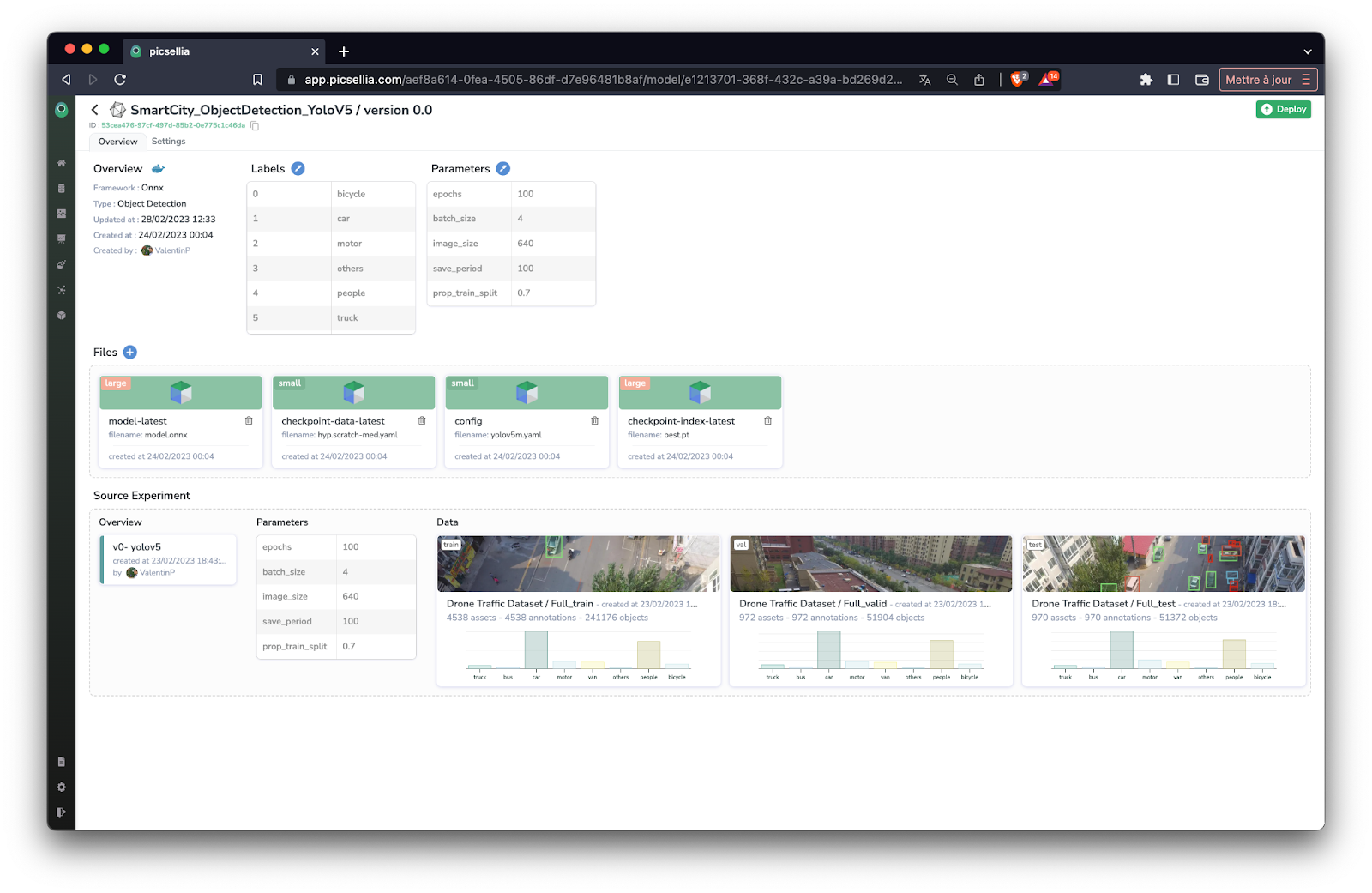
Ensuring traceability is also an extremely important thing to do. You want your newly hired engineers to be able to access any dataset, models and experiments in less than 3 clicks :)
The question: "where are the datasets?" is one of the most commonly asked questions inside organizations.
The most efficient way to make sure that no information ever gets lost is by always linking every training to their corresponding dataset and base architecture.
Build your knowledge base

The purpose of a knowledge base is to provide an organized, self-serve digital portal to quickly locate and access relevant information on a specific topic or field. It is also helpful for sharing and transferring knowledge as it can compile, organize, and communicate the necessary data to the intended audience.
A knowledge base can be structured in a variety of different ways, such as a database, digital library, or a document collection, with the end goal of creating a single source of data that is available to all users.
When it comes to Machine Learning, knowledge bases often take the form of registries, where you will find all the models that have been trained, and the Datasets your companies own.
To sum up, the on-boarding of data scientists and ML collaborators often ends up in a stressful and time-consuming process. These kinds of professionals require a tailored on-boarding process due to the complexity of ML teams, the fast evolving technologies and the dynamic opportunities the market keeps on creating. This is why an efficient onboarding must prioritize the traceability, reproducibility, and observability of tools and processes. In such a complex scenario, it is crucial that organizations are able to track their experiments, link their datasets to their training for traceability, and build a knowledge base in which both new and old employees are able to locate and access the relevant information they need.
A well-planned on-boarding strategy will save you and your teams a lot of time and money!