The ability to efficiently explore and manage vast datasets is crucial. Visual search technology is making it quicker and easier than ever. At its core, visual search allows users to explore massive image libraries, find similar images, and even identify specific features within a dataset. In this blog post, we'll dive into the capabilities of visual search, why it's important, and how it's transforming image data exploration.
What is Visual Search?
Visual search is a tool in Picsellia that allows users to query their datalake or a dataset of images based on either visual similarity with the image-to-image function, or based on specific text inputs with the text-to-image function.
Image-to-image search allows you to find all images similar to one sample image. Let's say you have images of a city, and you want to find and delete all the images of football fields. You take one image of a football field, and use it in the image-to-image search to find and delete all the similar images.
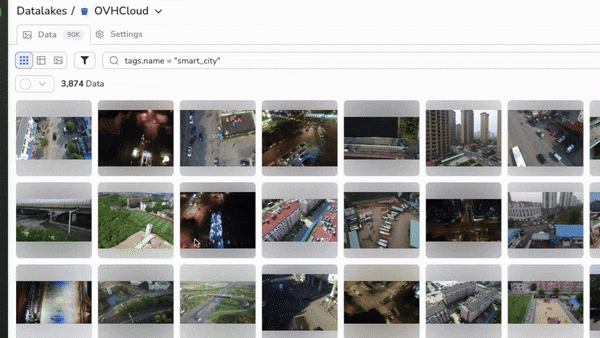
The other way to use visual search is by using specific text input in the text-to-image function. Maybe you want to find images that match a specific textual description like “night scenes”, “blurry images”, or “corrupted images” in order to clean your dataset.

Unlike traditional methods that rely on tags or metadata, our text-to-image search works without any predefined labels. As it’s based on an open-source implementation of OpenAI's CLIP (Contrastive Language-Image Pre-training), it connects visual concepts to natural language. This allows users to find images based purely on textual descriptions, like “blurry” or “night”. It’s a more flexible and efficient way that take data exploration to the next level.
Why Did We Create Visual Search?
The primary goal behind visual search is next-level data exploration. Many organizations today deal with immense datasets containing millions of images. Searching for a specific set of images in a dataset spanning multiple use cases can be tedious at best, and a nightmare at worst. Performing a simple query on such a dataset without the right tools would yield irrelevant results. But visual search, with its ability to include context metadata, makes searching more accurate.
Context Matters
A major breakthrough of the visual search feature is its ability to leverage context metadata. By narrowing searches to specific datasets, users can eliminate irrelevant results. For example, if your dataset spans multiple use cases (such as night photography for bird analytics or wind turbine inspections), visual search can refine the query to only the relevant subset. The images returned will fit the intended search purpose, thanks to the context metadata that attaches additional information, such as the dataset or use case, to each image.
This refined search process allows users to retrieve precise results and avoid the frustration of sifting through images from unrelated projects.
Key Use Cases
Data Cleaning
One of the most powerful uses of visual search is its ability to clean datasets. Let's say you're working on a dataset of images of wind turbines captured by drones. Occasionally, these drones might capture images of just the sky, which are irrelevant to the dataset's goals. Visual search can find all images that look like the sky, allowing you to remove them in a few clicks.
Finding Objects in Images
Another exciting use case is object detection. For instance, if you want to isolate all images with birds from a large dataset, visual search can identify images containing birds, even if they were uploaded for unrelated use cases (like wind turbine inspection). This feature is a game-changer for researchers working across multiple domains but needing to repurpose images for new tasks.

Text-Based Exploration
As previously mentioned, visual search can be driven by text input. You could type "blurry images," and the system will retrieve images that match this description. This feature is especially helpful for locating and removing low-quality or corrupted images from your dataset.
How Does Visual Search Work?
The power of visual search stems from the use of embeddings. Embeddings are like numerical fingerprints for images: they encapsulate visual features into vectors that can be compared with each other to determine how similar or different the images are. The visual search tool then compares these embeddings, clusters them, and allows users to query their datasets.
That's how, for example, in a dataset of wind turbine images, a user can search for images where the sky dominates the frame, helping to clean up the dataset by removing irrelevant content. Or, you could search for blurry images that may need to be excluded from a dataset. The visual search does this by recognizing these patterns in the embeddings.
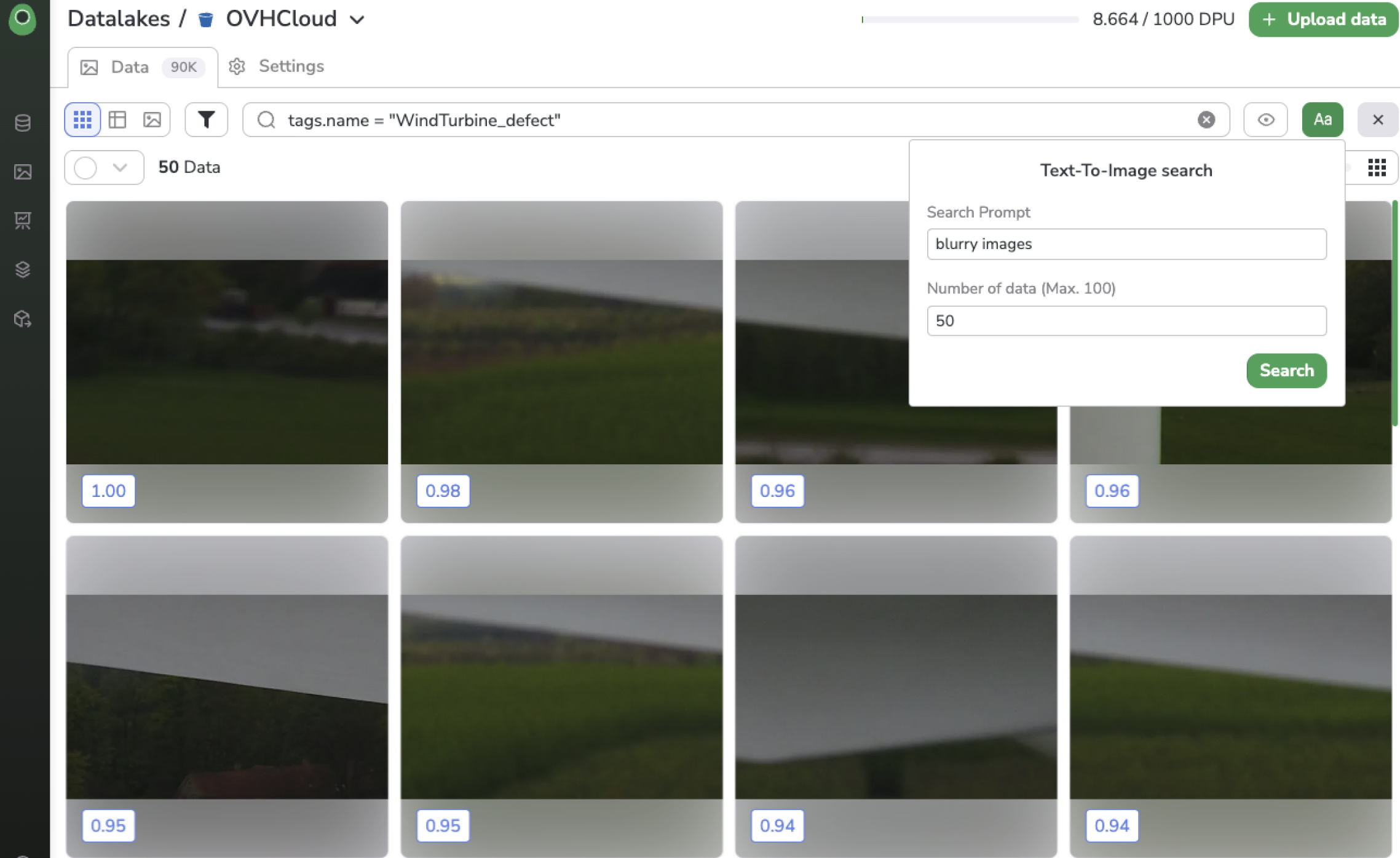
How is Visual Search Powered?
The current visual search model in use is OpenClip, a machine learning model developed by OpenAI. OpenClip is trained on image-text pairs, making it particularly effective for identifying correlations between visual data and text descriptions. However, it's important to note that OpenClip is trained on public domain data, meaning it may lack specialized knowledge for certain industries or use cases.
For example, while OpenClip can easily identify common objects like a bird or a banana, it might struggle with more specific terms - like a certain type of agricultural plant that isn’t part of its training data.
Future Developments: Specialized Models
As powerful as OpenClip is, there's a limitation when it comes to niche or industry-specific use cases. However, the team has an exciting solution in development. Future iterations of the visual search tool will include the ability to retrain the embedding model on a client’s own dataset. This will allow businesses to perform more accurate, domain-specific searches using natural language queries. Imagine an agriculture company that could search for specific crops or pests across their entire dataset with near perfect accuracy!
This addition to the search function is still in the works, but it's a promising next step that will make visual search even more powerful for industry-specific applications.
Conclusion
Visual search represents a massive leap forward in image data exploration. By making it easier to search, clean, and retrieve relevant data, this feature can save countless hours and help users extract deeper insights from their image datasets. With future updates on the horizon, including customized model training for specific domains, the possibilities for visual search are endless.
This powerful tool is a testament to how machine learning and smart data management can work together to transform how we interact with our data. Stay tuned for more updates as we continue to push the boundaries of what's possible in the world of visual search.